Venture capital (VC) has long been known for its reliance on human intuition and psychology in making investment decisions. However, as technology continues to improve and touch new highs, VC firms are increasingly turning to artificial intelligence (AI) and machine learning (ML) to enhance their investment processes. These new tools also help them in taking corrective measures and appropriate business-related decisions.
This blog explores the emergence of AI and ML in venture capital, the benefits they bring to the table, and how they are transforming the industry.
The Emergence of Data-Driven Investments
Traditional investment models often fall short due to their inability to account for real-world complexities and anomalies. This is where data-driven investments come into play in AI venture capital.
These investments, often derived from public web data, provide a more comprehensive and varied source of information for reviewing startups during their fundraising stage.
Public web data is a goldmine of information that offers unique insights.
For instance, consider the case of JCPenney in 2015. While traditional models predicted modest success, a big data company, RSMetrics, used satellite imagery of JCPenney’s parking lots to identify an increase in store traffic.
Investors who accessed this external data were ahead of the curve, capitalizing on the company’s unexpected performance.
How Machine Learning Is Used in Investment Decisions
Machine learning, a subset of AI, has become a crucial tool in the venture capital decision-making process. ML algorithms are designed to learn from data without the need for constant reprogramming, making them invaluable for sorting and analyzing large datasets efficiently.
Machine learning can predict future rewards by analyzing public web data. VC firms can leverage machine learning venture capital to assess the potential success of startups, making more informed investment choices.
Machine Learning in High-Frequency Trading
High-frequency trading (HFT) is another arena where machine learning plays a vital role. HFT involves rapid execution of a large number of orders within seconds, and AI is well-suited to process the vast amount of data involved in such trading.
Machine learning models can detect market price fluctuations and make precise bids, a task that would be impossible for humans to execute at such speeds.
Machine Learning and Signal Generation
ML-based algorithms have transformed signal generation, a critical aspect of investment decision-making. These algorithms can consider a wide range of data, including historical price patterns, volatility, sentiment analysis, price action, and more, to generate real-time trading signals.
Why Machine Learning is Important in Venture Capital
Machine learning provides the following key advantages to venture capital firms:
Unmatched Efficiency: ML algorithms can process vast amounts of data quickly and efficiently, curating long lists of startups that human brains would struggle to manage.
Overcoming Human Limitations: Humans have cognitive limitations when it comes to processing multiple factors simultaneously. AI and ML can handle complex analyses, taking into account both historical and future startup performance and market conditions.
Competitive Advantage: Implementing machine learning in venture capital can provide a significant competitive edge by improving decision-making, identifying better opportunities, and reducing human bias.
Venture Capital and Machine Learning Applications: Key Advantages
Finding Companies Seeking Funding: ML can filter startups based on specific criteria, helping VCs identify potential investment targets efficiently.
Identifying Opportunities: ML can detect early signs of growth in startups by analyzing indicators such as hiring plans and social media sentiment.
Timing and Investment: Machine learning can determine the ideal timing for investments by analyzing growth indicators and trends.
Growth Tracking: VCs can use ML to monitor the performance of their portfolio companies by analyzing online presence and sentiment data.
Employee Satisfaction Tracking: Machine learning can gauge employee satisfaction, a critical indicator of a company’s future growth potential.
Companies Utilizing AI and Machine Learning in VC
Several VC firms are already harnessing the power of AI and ML:
Correlation Ventures: Uses in-house ML models to analyze data from over 100,000 venture capital rounds to make investment decisions in under two weeks.
EQT Ventures: Utilizes an AI framework called “Motherbrain” to guide investment decisions based on proprietary data, startup financials, and more.
Sapphire Ventures: Considers using AI for making forecasts for portfolio companies, enhancing human decision-making with data-driven insights.
Hone: Partnered with AngelList to create a machine learning algorithm based on 30,000 deals, analyzing over 400 characteristics to identify top-performing startups.
Conclusion
The integration of venture capital machine learning into VC or Venture Capital is revolutionizing the industry. These technologies offer efficiency, data-driven decision-making, and a competitive edge that traditional methods cannot match. As the industry continues to evolve, machine learning will play an increasingly pivotal role in identifying and nurturing the startups that will shape the future.
Related read – Crafting the Perfect VC Pitch Deck: Your Key to Venture Capital Success
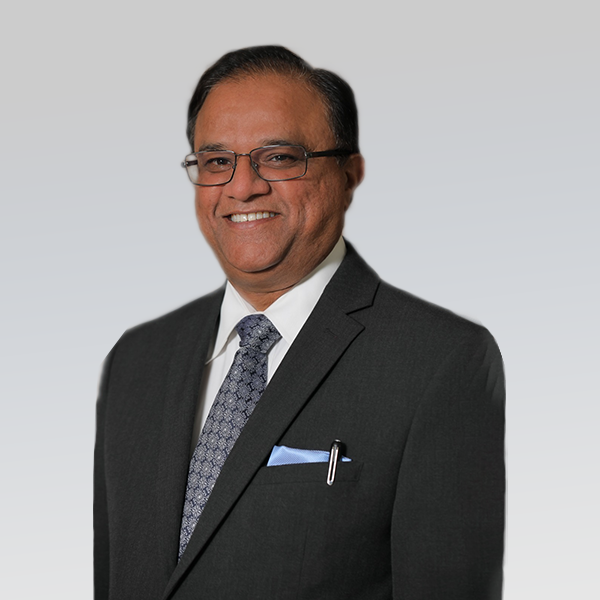
I’m the CEO of SPV Hub. Being a founder/ co-founder (of multiple businesses) and investor (in multiple startups) myself, I experienced the challenges that an investor and a founder face while raising capital and handling multiple deals. So, we created SPVHub to simplify everything related to SPV creation and management.
I am also the co-founder of Startup Steroid.